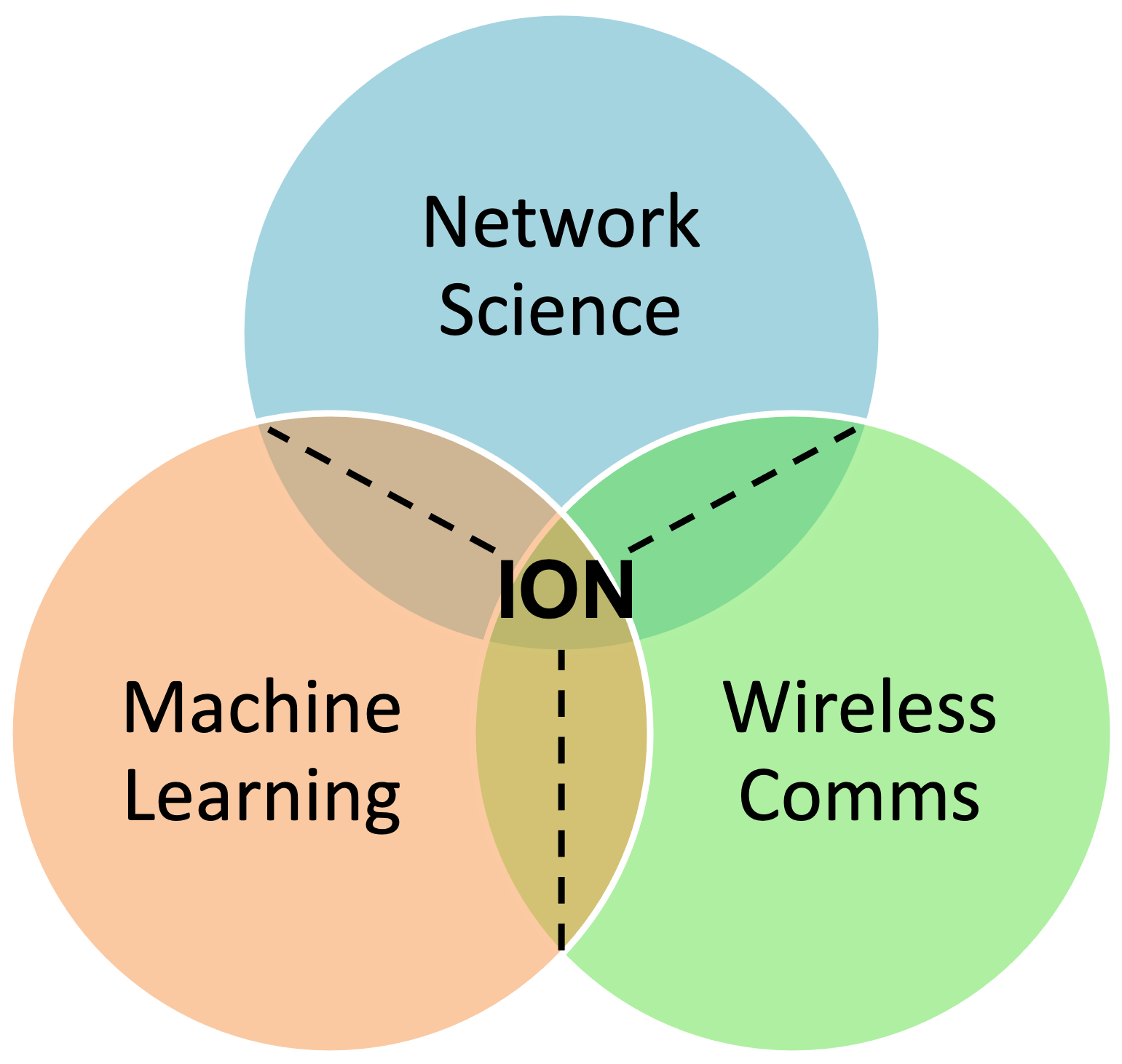
The Intelligence Optimization for Networks (ION) Lab at Purdue University conducts research at the intersection of network science/optimization and machine learning. This includes devising techniques for intelligence management in contemporary networked systems (i.e., networks for learning), and data-driven methodologies to optimize/defend how wireless networks operate (i.e., learning for networks).
ION produces methodologies spanning both communication network architectures (e.g., fog/edge computing, 5G/6G/NextG wireless, IoT) and certain social applications that run on top of these architectures (content delivery, online education, recommendation systems). Most of our efforts include significant theoretical (e.g., convergence analysis) and experimental (e.g., evaluations on real-world datasets) components.
The following summarizes a few key thrusts of ION's current research, with selected publications in each case. More publications can be found here.
T1: Federated Learning in Heterogeneous Networks
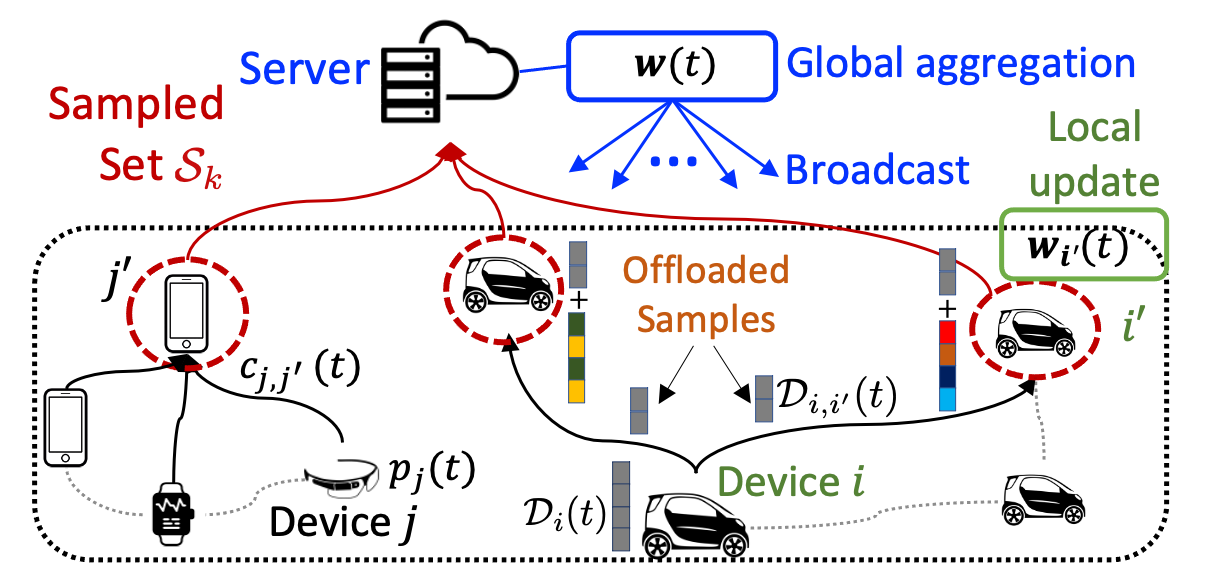
Federated learning has emerged as a popular technique for distributing the training of a machine learning model across a set of intelligent devices. In practice, these are often wireless edge/IoT devices, which are heterogeneous in their communication and computation resources, and tend to have diverse local dataset statistics. These heterogeneity factors must be carefully accounted for in optimizing the training process based on both learning quality and resource efficiency metrics.
Architecturally, we are considering how to migrate from the star topology of conventional federated learning to more distributed topologies that can manage these different dimensions of heterogeneity. In particular, we can blend the local update/global aggregation framework of federated learning with techniques such as intelligent device sampling (communication/computation heterogeneity) and direct device-to-device (D2D) communications (statistical diversity). We have developed new analytical results for model convergence based on these techniques, and employed them as the basis for control algorithms that optimize learning. Our experiments have demonstrated e.g., 50% faster convergence speed with a 3x improvement in resource efficiency.
Select/Recent Publications
- S. Azam, S. Hosseinalipour, Q. Qiu, C. Brinton. Recycling Model Updates in Federated Learning: Are Gradient Subspaces Low-Rank? International Conference on Learning Representations (ICLR), 2022.
- S. Hosseinalipour, S. Wang, N. Michelusi, V. Aggarwal, C. Brinton, D. Love, M. Chiang. Parallel Successive Learning for Dynamic Distributed Model Training over Heterogeneous Wireless Networks. IEEE/ACM Transactions on Networking, 2023.
- S. Wang, S. Hosseinalipour, C. Brinton. Multi-Source to Multi-Target Decentralized Federated Domain Adaptation. IEEE Transactions on Cognitive Communications and Networking, 2024.
T2: Optimizing Model Learning over Fog Networks
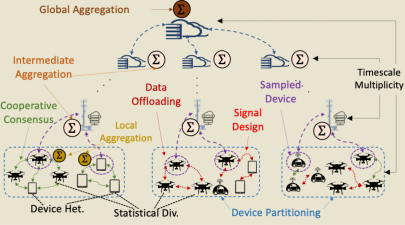
Another key challenge faced by existing distributed computing techniques is the volume and geographical span of edge devices. The central datacenter coordinating the computing task may be removed from the devices by several layers of a network hierarchy. Fog computing has recently emerged as an architecture for distributing network data processing tasks across this cloud-to-things continuum. Machine learning tasks require careful consideration in fog computing, especially when coupled with the challenges mentioned in the previous thrust.
To address this, we have been developing fog learning, a new paradigm for intelligently distributing machine learning across fog networks while accounting for heterogeneity and scalability properties. Fog learning aims to adapt device, fog, and cloud-level decision-making to jointly optimize model quality, service latency, and energy consumption metrics. In one case, we are considering how to augment federated learning with localized model aggregations throughout the fog hierarchy. To do so, we have experimented with novel local aggregation approaches via cooperative consensus formation within D2D-enabled local networks. Our resulting adaptive orchestration algorithms achieve the convergence speed of centralized training, with experiments demonstrating up to 50% reductions in energy consumption and service delay for the same model quality.
More information on our investigations into fog learning can be found at my NSF CAREER project webpage.
Select/Recent Publications
- F. Lin, S. Hosseinalipour, S. Azam, C. Brinton, N. Michelusi. Semi-Decentralized Federated Learning with Cooperative D2D Local Model Aggregations. IEEE Journal on Selected Areas in Communications, 2021.
- S. Hosseinalipour, S. Azam, C. Brinton, N. Michelusi, V. Aggarwal, D. Love, H. Dai. Multi-Stage Hybrid Federated Learning over Large-Scale D2D-Enabled Fog Networks. IEEE/ACM Transactions on Networking, 2022.
- D. Han, S. Hosseinalipour, D. Love, M. Chiang, C. Brinton. Cooperative Federated Learning over Ground-to-Satellite Integrated Networks: Joint Local Computation and Data Offloading. IEEE Journal on Selected Areas in Communications, 2023.
T3: Data-Driven Wireless Network Optimization
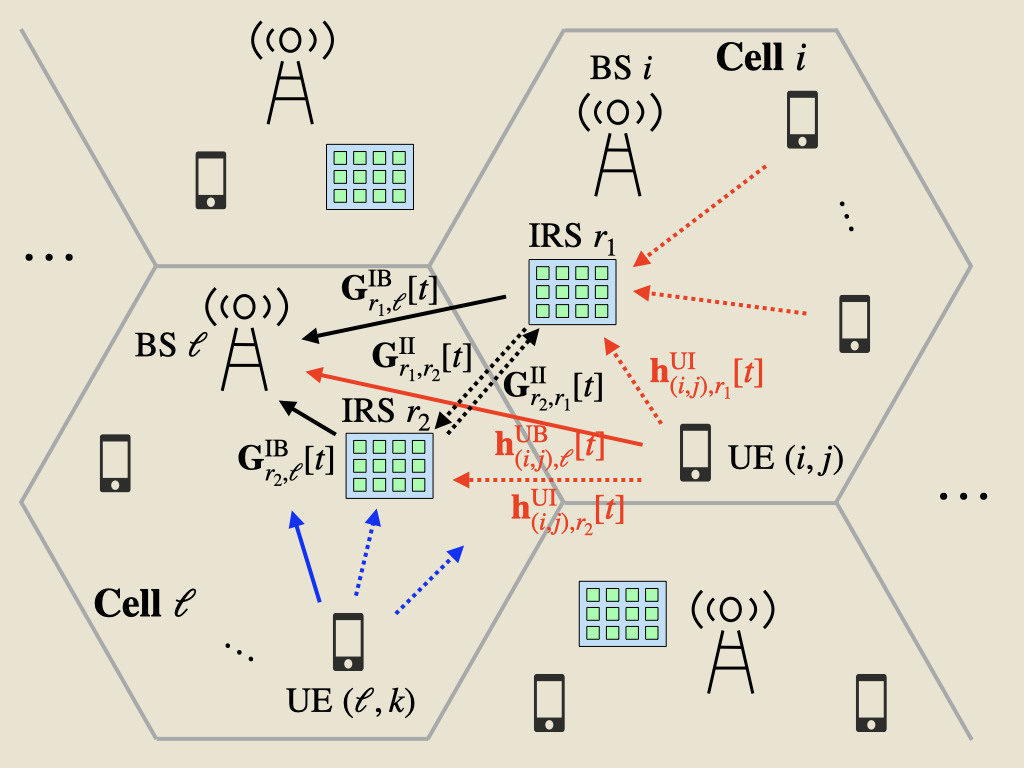
The convergence of today's wireless communication protocols with intelligent user applications is generating a plethora of data about network operation. The availability of these measurements presents a novel opportunity to re-examine existing network protocols from a data-driven perspective, and to develop intelligent protocols for NextG wireless services at the edge. While each use-case presents its own interesting questions, a few fundamental challenges exist: physical-layer measurement noise, input data size, and protocol delay sensitivity.
In investigating different use-cases we have been pursuing a cross-layer wireless learning approach, where learning of physical and link-layer processes takes place across the network protocol stack. In one line of work, we have been investigating wireless signal detection techniques, including building robustness to adversarial threats through adapting between time and frequency domain representations of input signals. Another direction of work has been considering MIMO beamforming design for communication and computation overhead minimization, leading to provably optimal beamformer strategies. Yet another direction has been considering configuration of intelligent reflecting surfaces (IRS) through multi-agent reinforcement learning techniques, so far yielding up to 35% improvements in uplink cellular data rates.
Select/Recent Publications
- J. Kim, S. Hosseinalipour, A. Marcum, T. Kim, D. Love, C. Brinton. Learning-Based Adaptive IRS Control with Limited Feedback Codebooks. IEEE Transactions on Wireless Communications, 2022.
- R. Sahay, M. Zhang, D. Love, C. Brinton. Defending Adversarial Attacks on Deep Learning Based Power Allocation in Massive MIMO Using Denoising Autoencoders. IEEE Transactions on Cognitive Communications and Networking, 2023.
- J. Kim, T. Kim, D. Love, C. Brinton. Robust Non-Linear Feedback Coding via Power-Constrained Deep Learning. International Conference on Machine Learning (ICML), 2023.
T4: Social Learning Network Personalization
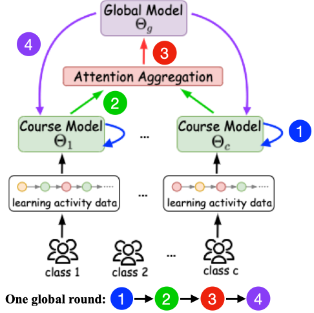
Contemporary social and communication networks are innovating when and how human learning takes place, as evidenced by the proliferation of online education during COVID-19. The fine-granular behavioral data (e.g., video-watching clickstream measurements) generated as users interact with online content and with one another in their social learning networks (SLN) presents an opportunity to optimize human learning with machine learning intelligence embedded in user devices. In doing so, we must also preserve the privacy of sensitive educational records.
We are developing learner modeling and content optimization methodologies that account for the multi-modal nature of behavioral data. In one direction, we are adapting our distributed learning tools from the prior thrusts to facilitate private and personalized learning analytics. This includes federated meta-learning methodologies that personalize user models based on identified or pre-existing student groups. Additionally, we have been developing statistical signal signal processing approaches for extracting knowledge-seeking behaviors from student clickstream sequences. Our multi-modal personalization methodologies consistently obtain 20% predictive gain over baselines, especially on underrepresented student subgroups. Large-scale experiments of our individualization system have shown statistically significant improvements in engagement and knowledge transfer.
Select/Recent Publications
- C. Brinton, S. Buccapatnam, L. Zheng, D. Cao, A. Lan, F. Wong, S. Ha, M. Chiang, H.V. Poor. On the Efficiency of Online Social Learning Networks. IEEE/ACM Transactions on Networking, 2018.
- Y. Chu, S. Hosseinalipour, E. Tenorio, L. Cruz, K. Douglas, A. Lan, C. Brinton. Mitigating Biases in Student Performance Prediction via Attention-Based Personalized Federated Learning. Conference on Information and Knowledge Management (CIKM), 2022.
- R. Sahay, S. Nicoll, M. Zhang, T. Yang, C. Joe-Wong, K. Douglas, C. Brinton. Predicting Learning Interactions in Social Learning Networks: A Deep Learning Enabled Approach. IEEE/ACM Transactions on Networking, 2023.
Current Personnel
Postdoctoral Research Associates
- Anindya Das
- Dong-Jun Han
- Jonggwang Kim
PhD Students
- Evan Chen
- Zhan-Lun Chang
- Yun-Wei Chu
- Tom Fang
- Guangchen Lan
- David Nickel
- Myeung Suk Oh
- Subham Saha
- Satyavrat Wagle
- Liangqi Yuan
- Shahryar Zehtabi
Masters Students
- James Brandewie
Undergraduate Student Researchers
- Joseph Lee
- Adam Piaseczny
Sponsors
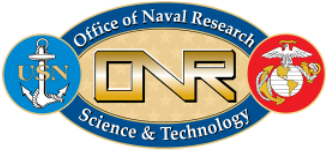
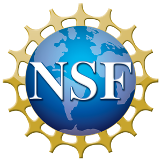
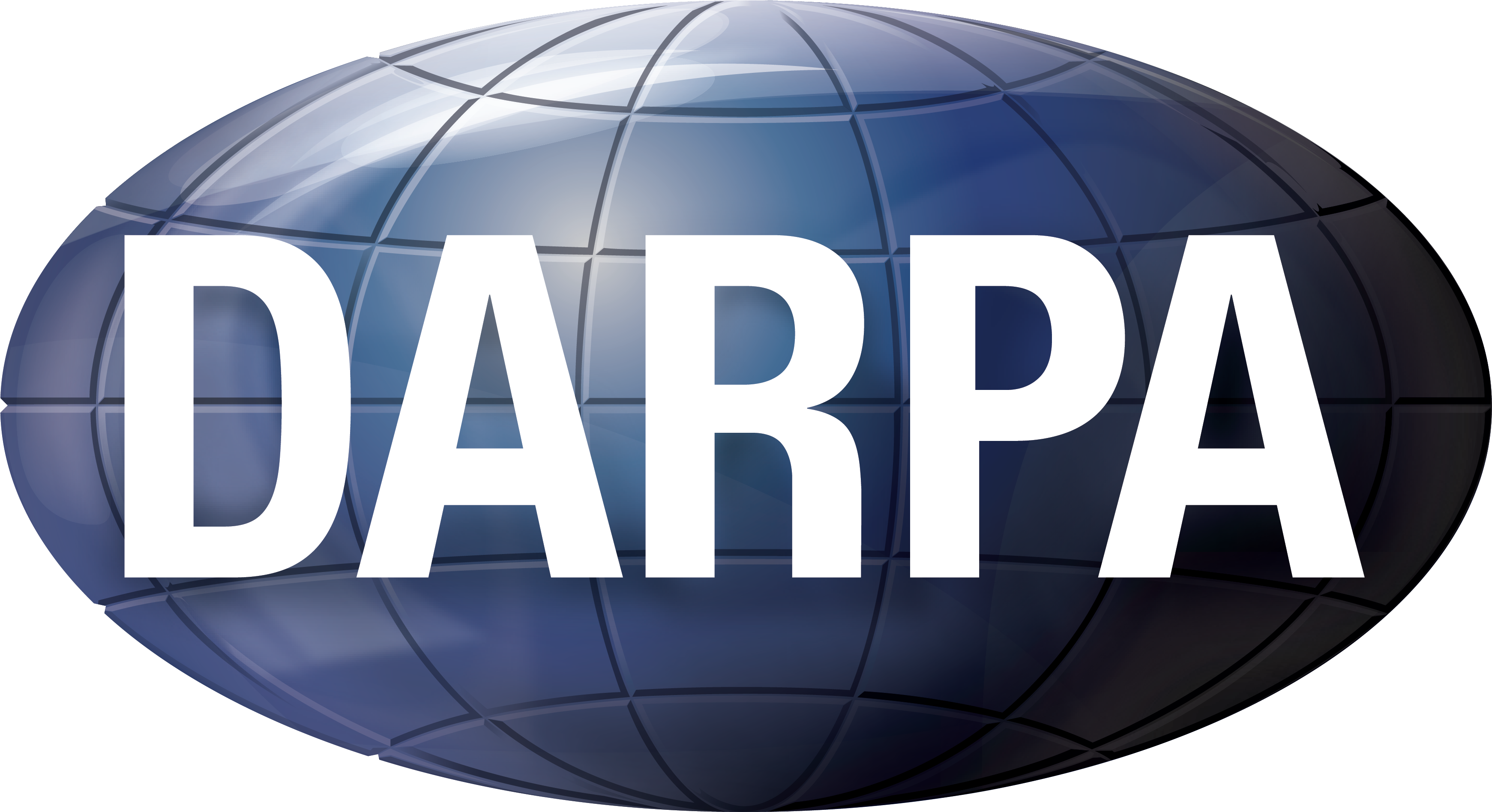
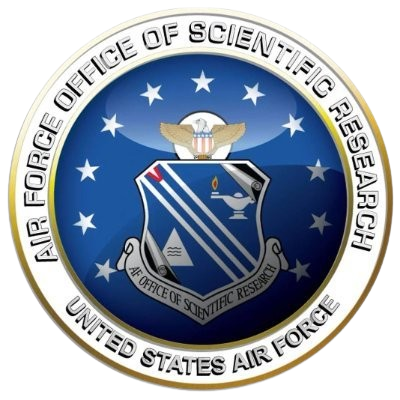
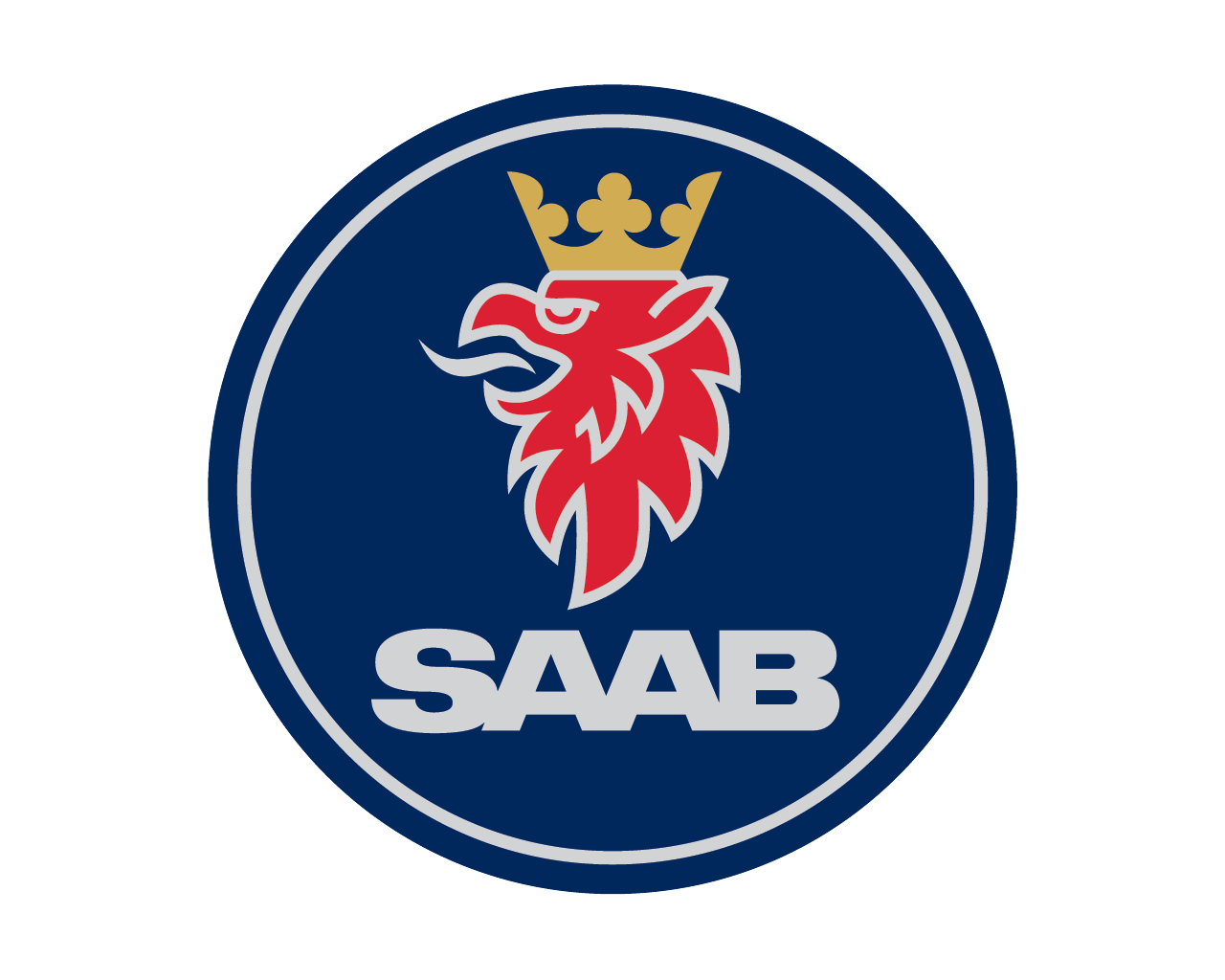
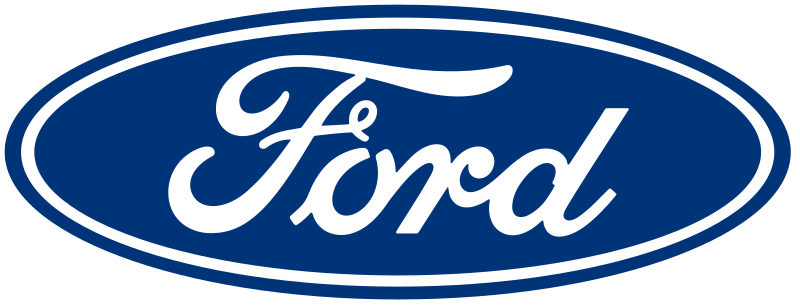
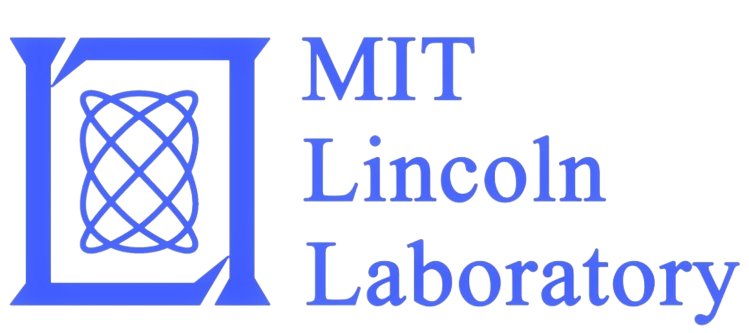
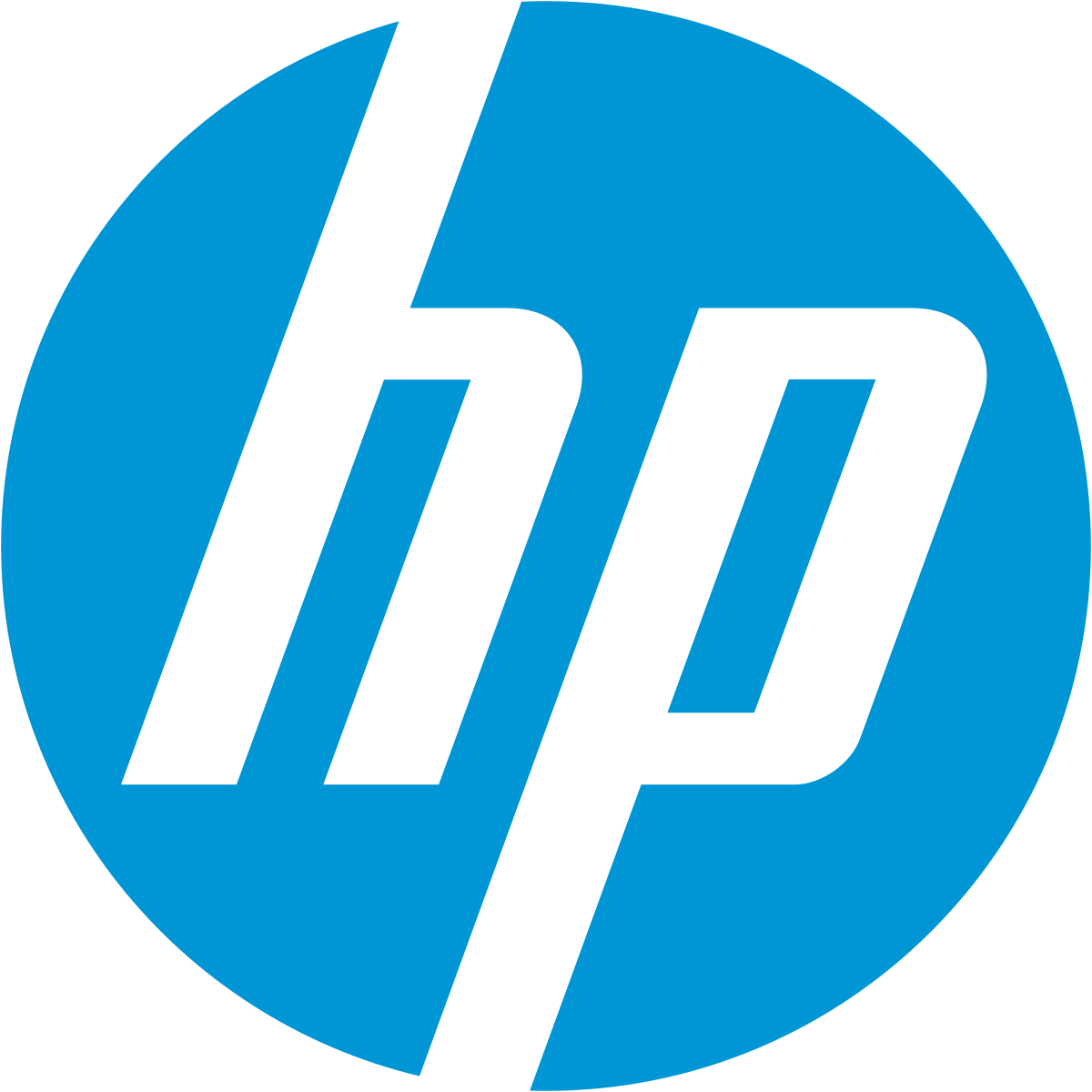
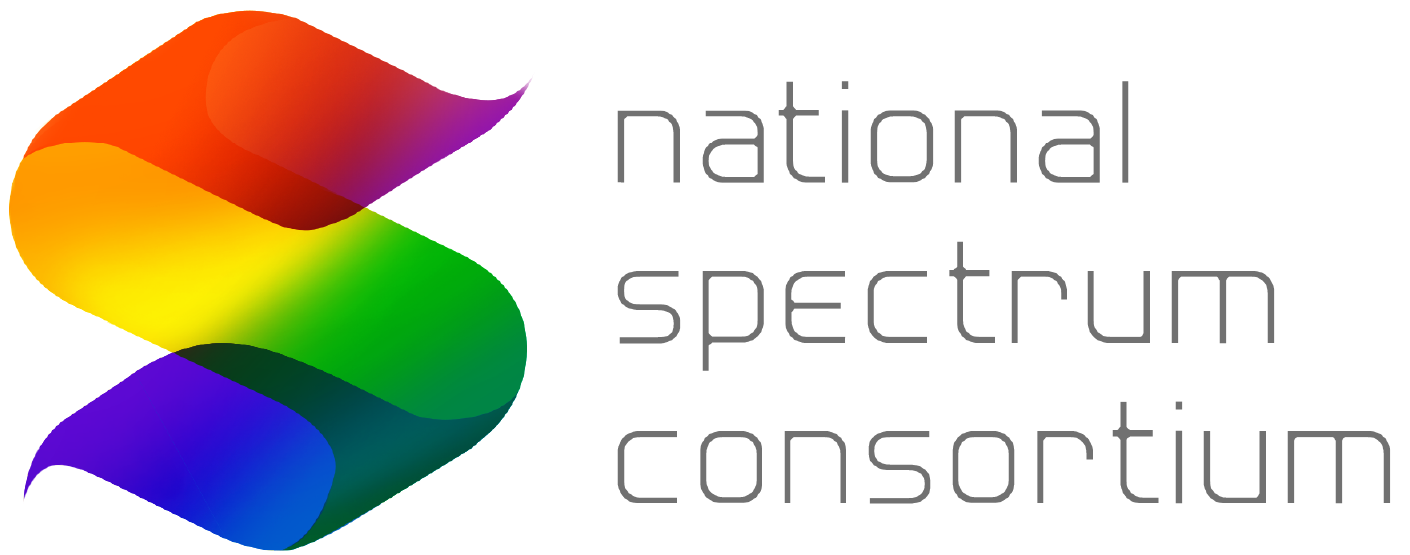
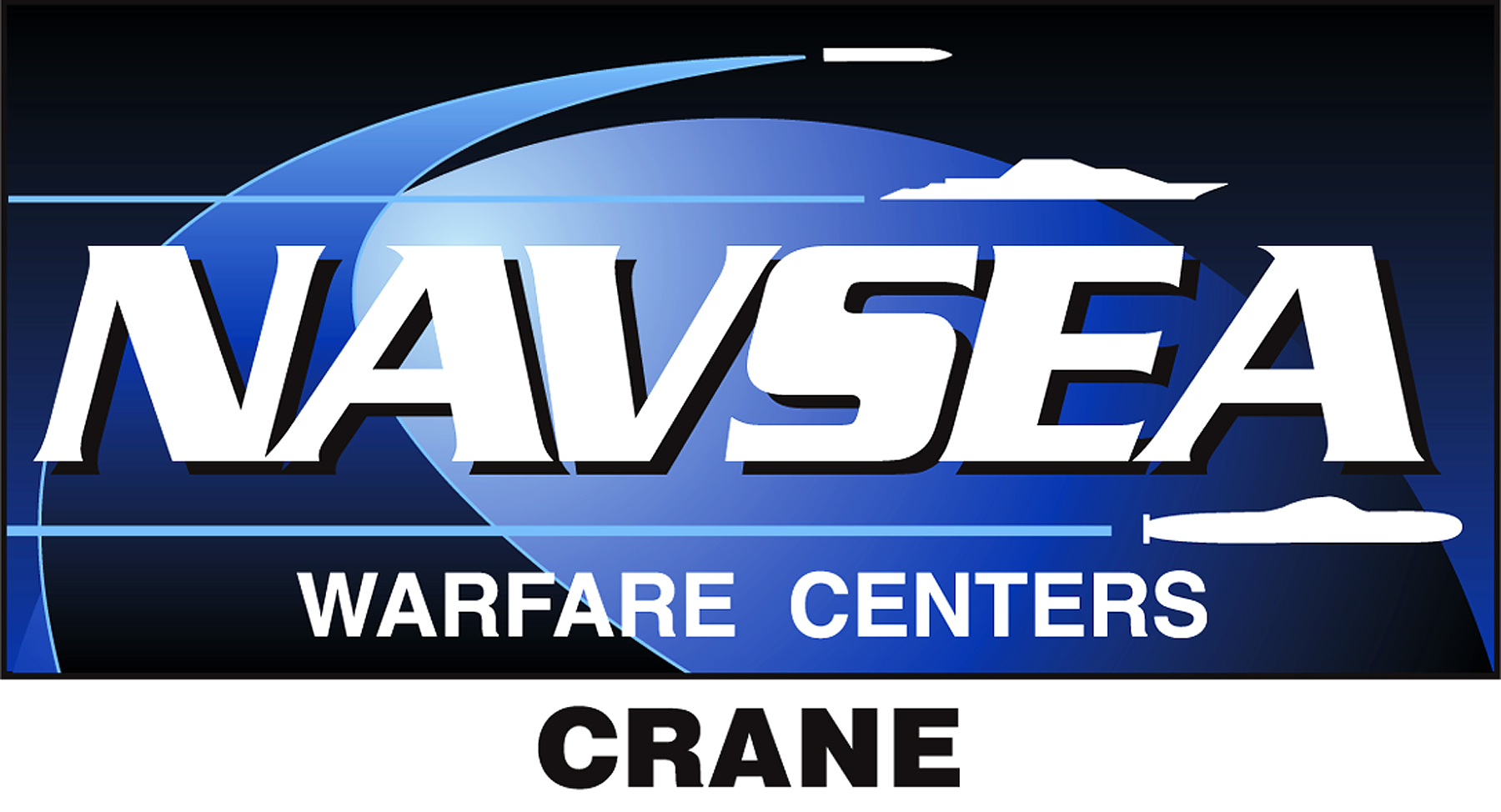
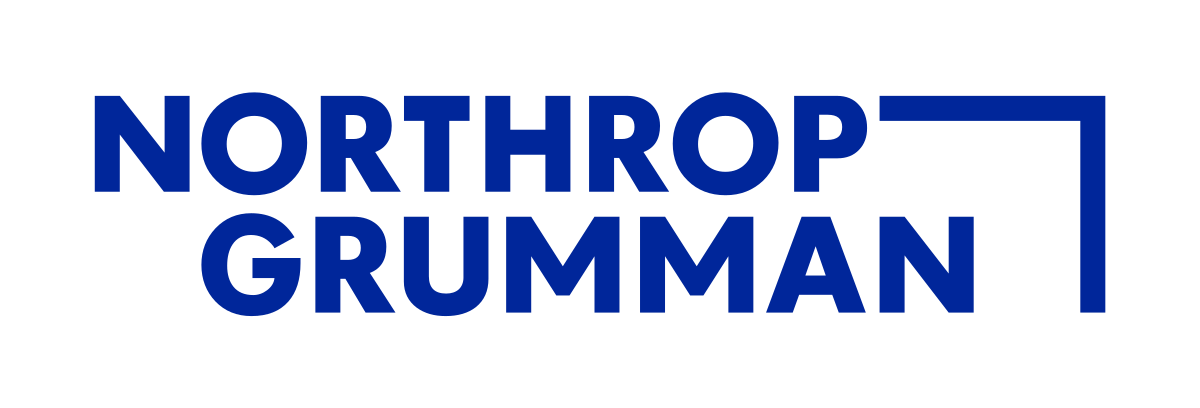
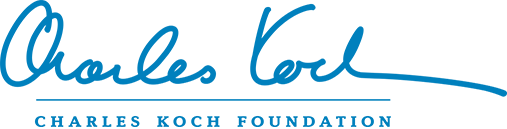